An introduction
Digital Twins are transforming our idea of innovation through a range of sectors including manufacturing, transportation, smart cities, and healthcare [1]. Recently, Gartner[2] stated that by 2021 half of large industrial companies will use Digital Twins and included them among the top 10 strategic technology trends. Despite this, the idea of Digital Twin is not new, as it was introduced by NASA[3] as “an integrated multi-physics, multi-scale, probabilistic simulation of a vehicle or system (…)” more than ten years ago.
As a matter of fact, the Digital Twin is a virtual replica of a physical system and it can include both the information embedded in its physical counterpart as well as models of physical components throughout the system’s lifecycle. Being based on a digital model of the assets, Digital Twins allow what-if analysis through simulations which can be run in real-time. In fact, a Digital Twin can collect data continuously from sensors and mutually pass information to its physical twin thanks to the IoT-enabling technologies, resulting in the synchronization with the field.
In the manufacturing sector, the Digital Twin is considered as a “virtual representation of a production system that is able to run on different simulation disciplines that is characterized by the synchronization between the virtual and real system, thanks to sensed data and connected smart devices, mathematical models and real time data elaboration[4]”.
Digital Twins have profound implications and enable new extraordinary capabilities. Their impact ranges from condition monitoring, which helps to generate previously unavailable insights to allow improvements in design and operations, to control applications, which enable to change assets’ functions or performances. Moreover, these innovations create new opportunities for optimization: algorithms can significantly improve performances, utilization, and uptime[5].
Hereafter, two of the research fields involving Digital Twins investigated in the I4.0Lab are presented. They have important industry-related applications compliant with the requirements of the fourth industrial revolution. Numerous are the industrial projects in which the I4.0Lab plays a central role for two reasons: on one side it acts as a prototype where innovative solutions are developed before being proposed and applied in real scenarios or as a testbed to implement the algorithms before their application in the funding companies.
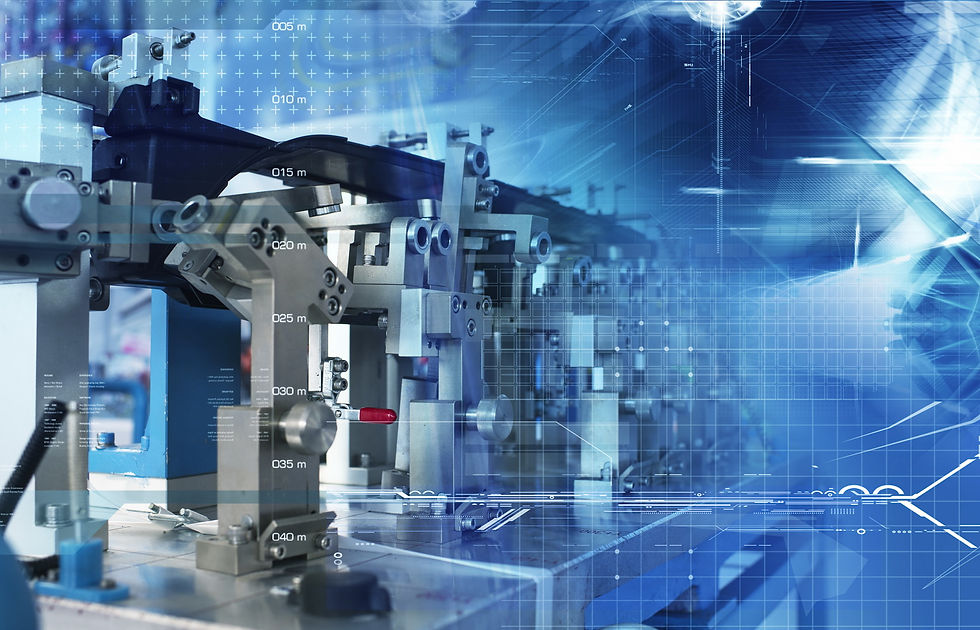
Digital Twin for scheduling
Scheduling activities cover a key-role in manufacturing processes. The challenges arising from different cases require to study, develop, and apply innovative methodologies to enhance the production capabilities of existing facilities. One of the focuses of the research group in the I4.0Lab is to develop scheduling optimization techniques for industries, merging theoretical results and practical needs. Topics of research currently under study include the design of frameworks to embed emerging technologies like Cyber-Physical Systems and Digital Twins, looking forward to achieving smart scheduling systems, able to integrate the knowledge coming from real-time data in the planning process. Other projects aim to optimize processes coming from different fields of application, showing that also traditional sectors propose interesting cases to experiment and improve state-of-the-art solutions. In fact, the peculiarities characterizing many scenarios impose requirements that push forward the group’s objectives in this field. Many projects involve the application of metaheuristic algorithms coupled with discrete event simulation. Currently one of these is focusing on systems characterized by the combination of no-wait constraints with flexible routing of jobs on multiple resources. Digital Twins provide a useful tool, as they enable to model the production system and simulate its behavior under varying conditions and with inputs coming from physical assets, with the aim to enrich modern scheduling systems with the capabilities offered by Industry 4.0.
Digital Twin for maintenance
Maintenance represents another fertile field where Digital Twin finds numerous applications in our I4.0Lab. Inspired by the ISO 13374 and the OSA-CBM framework[6], different projects are currently ongoing, each tackling one or more steps to develop a complete PHM (Prognostics and Health Management) process. More in detail, we are acting at different functional blocks of the process that goes from data acquisition to advisory generation. At the data acquisition step, projects are focused on overcoming data exchange and interconnection issues, guaranteeing data availability for further elaborations. Then, in data manipulation, the challenge is to enable automatic data cleaning, fusion, and contextualization so as the dataset be ready to be processed. Eventually, different projects are aiming at developing a complete DT that will encompass every functionality that may support the final decision-making, from state detection to novelty detection, integrated with real-time health indicators and Remaining Useful Life (RUL) prediction. Within these projects, all sorts of algorithms are explored and used, from more traditional statistical models towards advanced artificial intelligence ones. On top of this, a navigable dashboard could be created that boosts maintenance reactive and proactive actions on the machines. Finally, each result at any step is then integrated into an ever-growing DT solution that will cover and support the entire PHM process.
[1] Mark Purdy et al., “How Digital Twins Are Reinventing Innovation,” MIT Sloan Management Review, 2020, https://sloanreview.mit.edu/article/how-digital-twins-are-reinventing-innovation/. [2] Christy Pettey, “Prepare for the Impact of Digital Twins,” Smarter With Gartner, 2017, https://www.gartner.com/smarterwithgartner/prepare-for-the-impact-of-digital-twins/. [3] Mike Shafto et al., “DRAFT Modeling, Simulation, Information Technology & Processing Roadmap - Technology Area 11,” National Aeronautics and Space Administration, 2010, 27, https://www.nasa.gov/pdf/501321main_TA11-MSITP-DRAFT-Nov2010-A1.pdf. [4] Elisa Negri, Luca Fumagalli, and Marco Macchi, “A Review of the Roles of Digital Twin in CPS-Based Production Systems,” Procedia Manufacturing 11, no. June (2017): 939–48, https://doi.org/10.1016/j.promfg.2017.07.198. [5] Michael E. Porter and James E. Heppelmann, “How Smart, Connected Products Are Transforming Companies,” Harvard Business Review, 2015. [6] MIMOSA, Open System Architecture for Condition-Based Maintenance, Open Standards for Physical Asset Management, issued 2010.
Comments